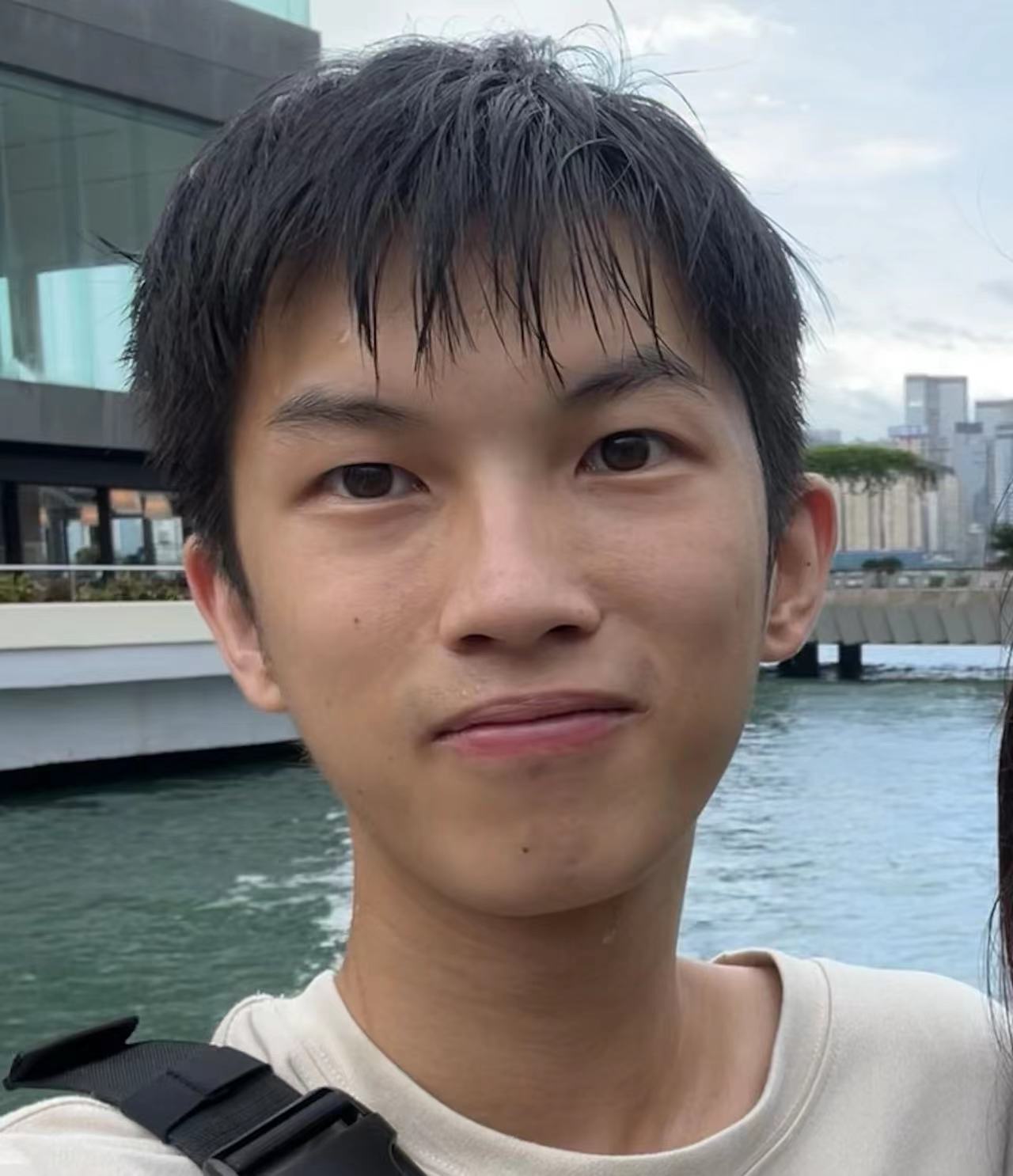
Zeping Ren
Hometown: Chongqing,China
Email:rzp22@mails.tsinghua.edu.com
Bio:
I am a master student studied in Tsinghua Shenzhen International Graduate School,
advised by Prof.Xiu Li.
I got my undergraduate degree in Department of Automation, Tsinghua University,
under the supervision of Prof.Yebin Liu.
My research is focus on 3D pose estimation and motion generation.